Redefining Information Retrieval: The Future of AI Search Technologies
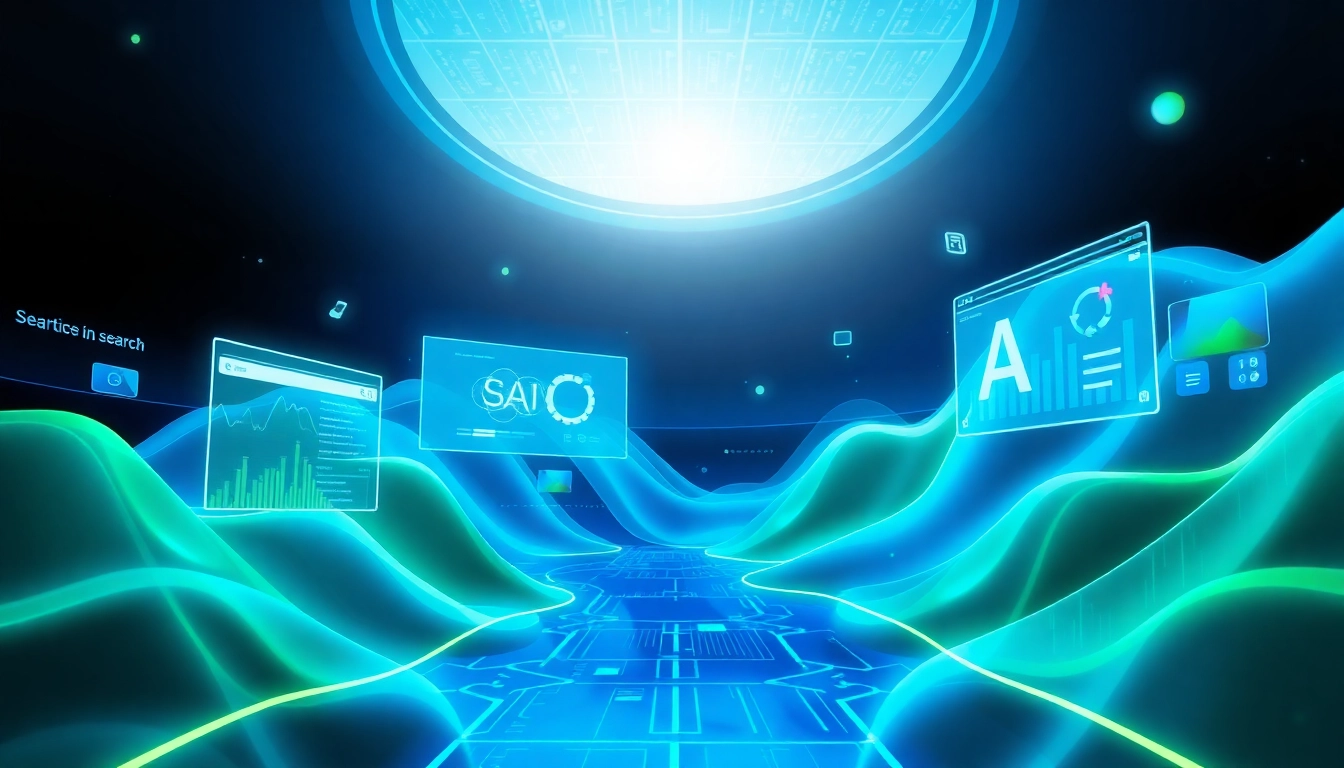
Understanding AI Search: An Overview
Artificial Intelligence (AI) is revolutionizing the way we seek and interact with information online. The emergence of AI search engines is reshaping user experiences, making information retrieval faster, more intuitive, and more relevant than ever before. This transformation is not just about improving search efficiency; it’s about integrating machine learning algorithms, natural language processing, and big data analytics to refine how searches return results. In this article, we delve deep into the world of AI search, exploring its essential components, current trends, best practices, challenges, and future innovations.
What is AI Search?
AI search refers to a digital search process enhanced by artificial intelligence techniques. Unlike traditional search engines that rely primarily on keyword matching and indexed page content, AI search leverages machine learning and natural language processing to understand the context and intent behind user queries. By doing so, AI search can deliver results that are more relevant and personalized, improving user satisfaction and engagement.
How AI Search Differs from Traditional Search
Traditional search engines operate primarily on defined algorithms that focus on keywords. When a query is made, the engine scans its indexed content for keywords, ranks pages based on SEO factors, and presents results accordingly. In contrast, AI search goes several steps further by interpreting user’s intent, analyzing context, and learning from previous interactions. This shift is pivotal; where traditional search can yield numerous links, AI search aims to present the most pertinent information in a concise and engaging manner.
Key Components of Effective AI Search Solutions
Several essential components contribute to the effectiveness of AI search engines:
- Natural Language Processing (NLP): NLP enables the AI to understand and generate human language, allowing it to process queries in a human-like manner.
- Machine Learning: Continuous learning algorithms improve search performance over time by feeding on user feedback and behaviors.
- Big Data Analytics: The capability to analyze vast datasets allows AI search engines to identify trends and patterns that enhance result relevance.
- Semantic Search: Semantic search focuses on the meaning of words and phrases to connect user intent with the most appropriate content.
Current Trends in AI Search Technologies
Emerging AI Search Engines
The landscape of AI search is rapidly evolving with several emerging contenders challenging established techniques. Notably, platforms like Google Gemini and Microsoft’s Copilot are innovating how people engage with information. These engines utilize AI-driven chat interfaces, making information retrieval a dynamic and interactive process. Such developments allow users to not just find information but also to query it further, obtaining results that are tailored to their specific needs.
Integration of Multimodal Search Capabilities
Multimodal search capabilities are a key trend shaping the future of AI search. By integrating various input styles—text, image, voice—AI search engines can process and analyze data from multiple sources. For example, Google’s AI Mode leverages image recognition alongside traditional text searches, enabling users to initiate searches based on visual cues. This integration is crucial for creating a more seamless search experience and caters to different user preferences.
Real-time Data Processing in AI Search
Real-time data processing allows AI search engines to deliver up-to-the-minute information. This is particularly essential for queries related to trending topics, news, or live events. By utilizing real-time data from social media, news outlets, and credible sources, AI search systems can present users with the most current and relevant information, making them more reliable than ever.
Best Practices for Implementing AI Search
Optimizing Content for AI Search Algorithms
Effective content optimization for AI search involves a shift from strict keyword-based targeting to a more nuanced approach that includes understanding user intent and context. Writers and marketers should focus on creating comprehensive, valuable, and relevant content. This includes utilizing structured data markup to help search engines better comprehend the content’s context and relevance.
Leveraging User Data for Improved Results
User data plays a crucial role in refining AI search algorithms. By analyzing search patterns, preferences, and feedback, AI systems can tailor results that align more closely with user expectations. Collecting and responding to user interaction data helps improve the precision and reliability of future searches, leading to enhanced user satisfaction and loyalty.
Continuous Learning and Adaptation in AI Search
Continuous learning is a hallmark of effective AI search. Algorithms should be designed to learn and evolve based on user interactions. For instance, if a user consistently prefers certain types of results over others, the system should adapt and prioritize similar content in future searches. Implementing a feedback loop where user choices inform future results is key to maintaining relevance and efficiency.
Challenges and Considerations in AI Search
Data Privacy Issues with AI Tools
As AI search engines evolve, issues surrounding data privacy remain a significant concern. With the collection of vast user data comes the responsibility to protect this information. Implementing stringent data privacy policies, obtaining user consent, and being transparent about data usage are essential practices to maintain user trust while leveraging data for personalization.
Citation Accuracy in AI Search Results
Citation integrity is another major challenge facing AI search technologies. Many AI engines struggle with accurately citing sources, leading to potential misinformation. Ensuring that AI systems provide verifiable sources and citations is critical to maintaining credibility. This includes developing algorithms that can trace back information to reliable sources, particularly in news and academic contexts.
Addressing Bias in AI Algorithms
Algorithmic bias is a prominent issue within AI search technologies. Bias in training data can lead to skewed search results, which may reinforce stereotypes or exclude certain demographics. To counteract this challenge, developers must employ diverse and representative datasets for training AI models while continuously testing algorithms for fairness and bias correction.
The Future of AI Search Technologies
Predictions for AI Search Innovations
Looking ahead, the future of AI search will likely involve more advanced algorithms that prioritize personalization, contextual understanding, and seamless integration across platforms. Innovations such as emotion-aware search systems that detect user sentiment and adjust outcomes accordingly may become commonplace. Furthermore, the integration of virtual reality (VR) and augmented reality (AR) with AI search is anticipated to enhance immersive information retrieval experiences.
Impact on User Experience and Information Access
As AI search continues to evolve, the user experience is expected to become increasingly intuitive, making access to information simpler and more engaging. Seamless interactions across devices and platforms will empower users to access information effortlessly, influencing how learning, shopping, and leisure activities are conducted. Users will experience greater control and customization in their search processes, leading to a more tailored and satisfying interaction with technology.
Potential Ethical Concerns and Resolutions
Ethical concerns surrounding AI search will need to be addressed as these technologies become widespread. Potential issues include questions about surveillance through data collection and the implications of AI making decisions for users. Creating ethical frameworks, conducting regular audits, and ensuring accountability in AI development will be crucial in curbing these issues while protecting user privacy and freedom of choice.
Leave a Reply