Essential Steps for How to Hire a Hacker Safely and Effectively
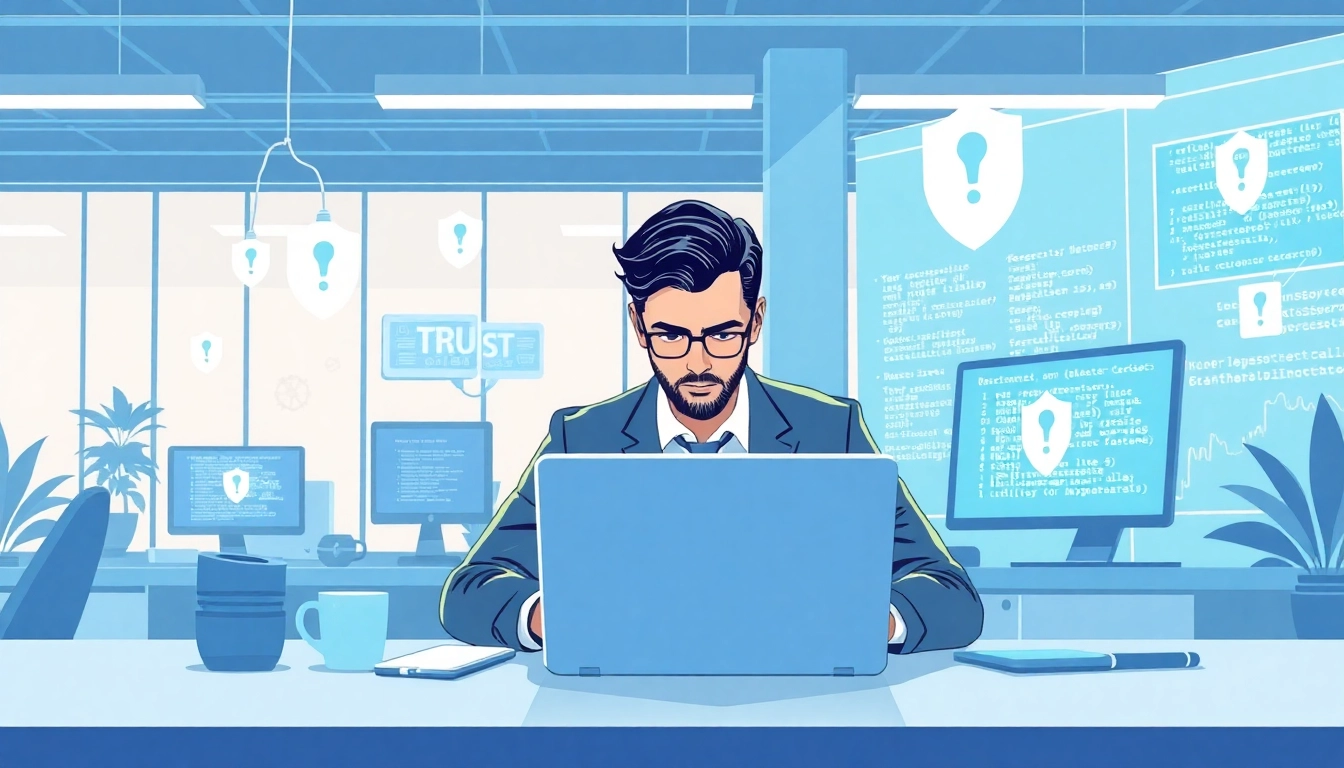
Understanding the Role of a Hacker
In today’s digital landscape, understanding the role of a hacker is essential for both individuals and organizations looking to enhance their cybersecurity posture. While many people associate hackers solely with malicious activities, the reality is far more nuanced. From ethical hackers who test systems for vulnerabilities to malicious actors who exploit weaknesses for personal gain, the hacker community is diverse. This article aims to guide you on how to hire a hacker safely and effectively, ensuring you find the right expertise for your needs.
1. Types of Hackers: Ethical vs. Malicious
Before you embark on the journey of hiring a hacker, it’s critical to understand the different types that exist:
- Ethical Hackers: Also known as white hat hackers, they use their skills to help organizations identify and mitigate security vulnerabilities. They operate legally and with the permission of the organizations they are testing.
- Malicious Hackers: These individuals, often referred to as black hat hackers, exploit vulnerabilities for personal gain, such as theft or disruption. Engaging with them can lead to significant cybersecurity issues.
- Gray Hat Hackers: These hackers fall somewhere in between; they may operate without permission but do not have malicious intent. They might disclose vulnerabilities to the affected organization after exploitation.
Understanding these distinctions will help you clarify your needs and objectives when seeking to hire a hacker.
2. The Skills to Look For
When hiring an ethical hacker, consider the following essential skills:
- Technical Proficiency: A deep understanding of programming languages, networking, and operating systems is crucial.
- Certifications: Look for valid certifications such as Certified Ethical Hacker (CEH), Offensive Security Certified Professional (OSCP), or CompTIA Security+.
- Analytical Thinking: The ability to think critically and troubleshoot complex problems is vital in identifying security vulnerabilities.
- Communication Skills: A hacker must effectively communicate findings to both technical and non-technical stakeholders.
3. Common Misconceptions about Hiring Hackers
There are several myths surrounding the hiring of hackers that can lead to misconceptions:
- All Hackers Are Criminals: Many believe that all hackers have malicious intent, but ethical hackers provide essential services to protect against cyber threats.
- Hiring a Hacker Is Only for Big Corporations: In reality, small businesses and individual users also benefit from hiring ethical hackers to secure their systems.
- It’s Always Expensive: The cost of hiring a hacker can vary widely; there are affordable options available based on your specific needs and budget.
Identifying Your Needs
Identifying your specific needs is a crucial first step in the hiring process. Understanding what you require from an ethical hacker will streamline the selection process and help you choose the right candidate.
1. Defining Objectives: What Do You Need?
Clearly define your objectives before hiring a hacker. Common goals include:
- Pentest (Penetration Testing): Assessing the security of your systems by simulating an attack.
- Vulnerability Assessments: Identifying and prioritizing vulnerabilities within your network.
- Incident Response: Assisting your team when a breach occurs and mapping out how to respond effectively.
By establishing your objectives, you can better communicate your needs to potential candidates, ensuring they can meet your expectations.
2. Assessing Security Vulnerabilities
Performing an initial assessment of your organization’s security vulnerabilities can provide clarity on what services you may require. Look at aspects like:
- Network Security: Examine firewalls, intrusion detection systems, and network configurations.
- Application Security: Assess your web applications for potential flaws.
- Data Security: Evaluate how sensitive data is stored and protected in your systems.
A thorough understanding of your vulnerabilities will allow a hacker to focus on the areas that need improvement.
3. Establishing a Budget for Services
Budgeting is a critical aspect of the hiring process. Service costs can depend on several factors:
- The complexity of the task: More complicated security assessments may require extensive manpower and specialized skills.
- The experience of the hacker: Seasoned professionals typically charge more due to their expertise and proven track record.
- The duration of the engagement: Some services may require ongoing relationships, impacting the budget.
Prioritize your needs and look for an affordable solution that does not compromise on quality.
Where to Find Hackers
Your next step is finding the right platform or avenue to hire an ethical hacker. Here are some effective approaches:
1. Online Marketplaces and Platforms
Various online platforms cater specifically to the hiring of ethical hackers and cybersecurity professionals:
- Freelance Websites: Platforms like Upwork, Toptal, and Guru allow you to post jobs and review candidates extensively.
- Specialized Services: Websites that provide ethical hackers for specific tasks often have vetted professionals to choose from. These include HackerOne and Bugcrowd.
Using these platforms can streamline your search and provide access to a wide range of candidates.
2. Networking and Referrals
Networking remains one of the most effective ways to find trusted hackers. Consider:
- Seeking referrals from peers: Ask within your professional network for recommendations.
- Attending Industry Conferences: Events such as Black Hat and DEF CON provide opportunities to meet hackers and experts in person.
- Joining Professional Associations: Membership in groups like ISC² or ISACA can connect you with qualified individuals.
3. Social Media and Community Groups
Social media platforms and community forums are an excellent way to find hackers. Platforms such as LinkedIn and Reddit often have groups or threads related to cybersecurity where you can find recommendations and solicit help.
Engaging in discussions can lead to valuable contacts in the hacker community.
Evaluating Candidates
Once you have identified potential candidates, the evaluation process is critical in ensuring you hire the right hacker. Here’s how to effectively assess candidates:
1. Reviewing Credentials and Previous Work
Verify the hacker’s credentials and previous work to ensure they possess the necessary expertise:
- Check certifications and qualifications: Ensure they have relevant certifications and training in ethical hacking.
- Review case studies or testimonials: Look for documented success stories or testimonials from past clients.
2. Conducting Interviews and Assessments
Interviews can provide insight into a candidate’s thought process and problem-solving abilities. Consider incorporating:
- Technical interviews to discuss scenarios and gauge their problem-solving skills.
- Practical assessments, such as tests or simulations, to evaluate their hands-on abilities.
3. Checking References and Reviews
Finally, validate the credibility of the hacker by checking references from previous clients. Request insights on the hacker’s performance, reliability, and professionalism.
Finalizing the Hire
Once you have selected a candidate, it’s essential to finalize the engagement correctly to avoid future misunderstandings.
1. Drafting a Clear Contract
Ensure that a comprehensive contract is in place that outlines:
- Scope of Work: Define the exact tasks, deadlines, and deliverables expected from the hacker.
- Payment Terms: Clearly outline payment expectations, including milestone payments if applicable.
- Confidentiality Clauses: Protect your sensitive information by including non-disclosure agreements.
2. Establishing Communication Channels
Set clear communication channels that facilitate smooth collaboration. Tools like Slack, Trello, or email should be utilized to keep all parties informed throughout the engagement.
3. Setting an Evaluation Plan for Outcomes
Establish metrics to evaluate the success of the hacker’s work. This could include:
- Assessing the number and severity of vulnerabilities identified.
- Monitoring improvements in system security over time.
Having clear performance metrics will help ensure the hacker meets the established goals and objectives.
Conclusion
Hiring a hacker can be a crucial decision for individuals and businesses seeking to bolster their cybersecurity. By understanding the types of hackers, identifying your security needs, finding the right candidates, and conducting thorough evaluations, you can ensure a successful hiring process. With the right ethical hacker in place, you can better protect your systems from potential threats and vulnerabilities, paving the way for a more secure digital experience.