Choosing the Right AI Checker: A Guide to Analyzing AI-Written Content
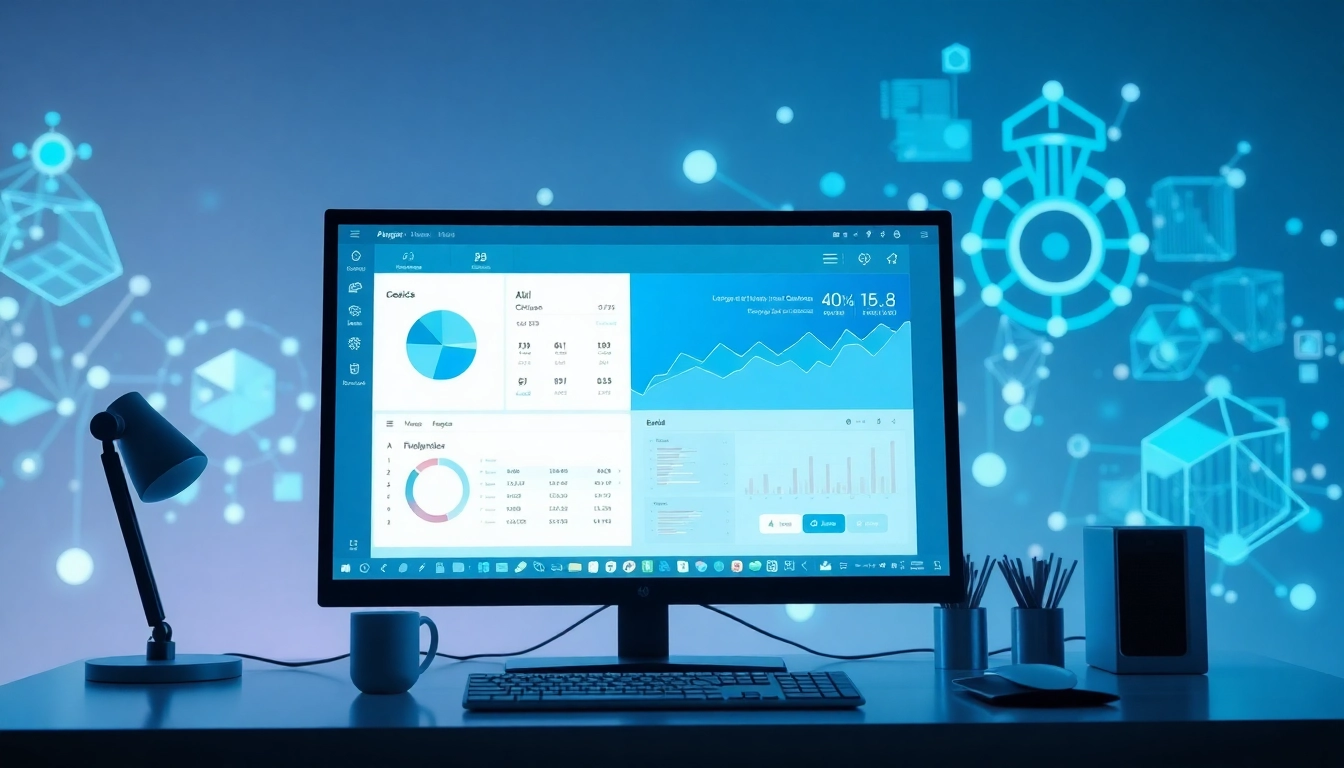
Introduction to AI Checkers
In an era where artificial intelligence seamlessly integrates into our daily lives, distinguishing between human-written and AI-generated text has become increasingly critical. The emergence of ai checker tools plays an essential role in this differentiation, providing users with the means to determine the origin of written content. These tools have garnered significant attention due to the rise of sophisticated AI text generators, such as ChatGPT, GPT-3, and others, which can produce convincing and coherent narratives and articles.
Understanding AI-Generated Content
AI-generated content refers to text or narratives created by artificial intelligence models using automated processes rather than human authorship. Various language models rely on vast datasets to learn language patterns, grammar, and context, enabling them to generate text that often mirrors human writing closely. This can include news articles, poetry, marketing content, and academic papers. As beneficial as these tools are, they pose challenges regarding authenticity, ethics, and potential misinformation.
What is an AI Checker?
An AI checker is a tool designed to analyze text and ascertain whether it was produced by a human or an AI. These tools utilize various methodologies to assess the structure, phrasing, and coherence of the text. By employing algorithms that look for patterns characteristic of AI writing, AI checkers can provide insights into the content’s origin. This technological advancement aims to help educators, businesses, and individuals ensure content authenticity and uphold academic integrity.
Importance of AI Detection
The importance of AI detection cannot be overstated. As AI writing tools become more advanced, the potential for misuse—especially in academic and professional settings—grows correspondingly. AI detection tools help combat this issue by:
- Preserving Academic Integrity: Schools and universities are increasingly concerned about students submitting AI-generated assignments as their own work.
- Ensuring Content Authenticity: Businesses need to verify that their content is genuinely authored and not AI-generated, maintaining integrity in marketing and branding.
- Combatting Misinformation: In an information-rich age, distinguishing credible content from AI-generated misinformation is crucial for informed public discourse.
Types of AI Checkers Available
Free vs. Premium AI Checkers
AI checkers are broadly categorized into free and premium versions, each with its distinct advantages and limitations.
- Free AI Checkers: These tools provide essential detection functionalities without any cost. Users may find features such as basic compatibility checks, simple user interfaces, and limited text input lengths. However, the depth and sophistication of analysis often pale in comparison to premium options.
- Premium AI Checkers: These services generally offer more advanced features, including comprehensive reporting, extensive text evaluation, and integration with other platforms. Premium AI checkers may also provide insights into content cohesiveness and suggest improvements based on detected anomalies.
Comparison of Popular AI Checkers
When evaluating different AI checkers, users should consider the features, accuracy, pricing, and user feedback. Here is a brief overview of a few popular options in the market:
- ZeroGPT: Known for its advanced detection capabilities, it utilizes a multi-stage methodology to identify AI-generated text.
- QuillBot: This detector provides free AI detection, carefully assessing content generated from tools like ChatGPT and GPT-4.
- Grammarly AI Detector: Apart from grammar checking, Grammarly also offers AI detection features, making it a versatile tool for writers.
- GPTZero: Promoted as one of the most advanced options, it has received widespread media coverage for its effectiveness in distinguishing AI content.
Key Features to Look For
When choosing the right AI checker, consider the following essential features:
- Accuracy: A reliable detection rate is paramount. Look for tools that provide proof of results through user testimonials or comparative studies.
- User Interface: An intuitive interface is essential for ease of use, ensuring users can navigate the platform without frustration.
- Reporting Capabilities: Detailed reports that explain the analysis and offer feedback on how to enhance the detected content can be valuable.
- Integration Options: Integration with content management systems (CMS) can streamline workflows and enhance productivity.
How to Effectively Use an AI Checker
Step-by-Step Guide to Using AI Checkers
Using an AI checker may vary slightly depending on the specific tool, but the general process is similar. Here’s a step-by-step guide:
- Copy Your Text: Begin by selecting and copying the text you wish to analyze.
- Access the AI Checker: Navigate to the website of your chosen AI checker.
- Paste Your Text: In the designated field, paste the copied text for analysis.
- Initiate the Analysis: Click the “Check” or “Analyze” button to commence the evaluation.
- Review the Results: Analyze the results provided by the checker, including the percentage of AI detection and any specific indicators noted.
Tips for Accurate Analysis
To maximize the accuracy of analyses from AI checkers, consider these best practices:
- Use Diverse Text Samples: Checking a variety of text lengths and styles can help verify the checker’s reliability across different content types.
- Avoid Over-Simplistic Text: Submitting overly simplistic or formulaic text may lead to misleading detection results.
- Cross-Reference Results: Consider using multiple AI checkers to confirm findings and achieve a comprehensive understanding of your content’s AI likelihood.
Interpreting Results from AI Checkers
After the analysis, interpreting the results is crucial for understanding the implications of the findings. Most checkers provide:
- AI Detection Percentage: A numerical value indicating the likelihood that the content is AI-generated.
- Highlighted Sections: Specific areas that triggered the AI detection flag can offer insights into what changes to make.
- Recommendations: Follow-up suggestions to refine the existing text, potentially improving human readability and reducing AI-like characteristics.
Challenges in AI Detection
Common Limitations of AI Checkers
While AI checkers serve a vital function, they come with certain limitations:
- False Positives: AI checkers might mistakenly categorize human-written content as AI-generated, particularly if it follows common phrasings and structures.
- Inconsistencies Across Tools: Different AI detection tools may yield varied results based on their algorithms, which can lead to confusion for users.
- Dependence on Training Data: The effectiveness of an AI checker often hinges on its underlying training data; models trained on limited datasets may struggle with novel or nuanced writing styles.
How to Address Detection Failures
To mitigate the consequences of detection failures, users can:
- Revise Text Structures: Vary sentence structures and word choices to make content less predictable and harder to classify as AI-generated.
- Utilize Human Review: Employ the analysis of a human content editor to supplement results from AI checkers, ensuring a comprehensive evaluation.
Future Trends in AI Detection Technology
The future of AI detection may be characterized by several emerging trends:
- Increased Accuracy: As AI technology evolves, so too will detection methodologies, leading to higher precision in content assessment.
- Integration of Machine Learning: Advanced AI checkers will increasingly use machine learning algorithms to continuously adapt and improve upon their detection capabilities.
- Focus on Ethical Standards: The growing conversation around ethics in AI will influence how AI checkers operate, ensuring they support transparency and accountability.
Conclusion and Recommendations
Best Practices for Choosing an AI Checker
When selecting an AI checker, consider these best practices:
- Research Options Thoroughly: Investigate features, user reviews, and trial options to find a tool that best suits your needs.
- Prioritize Reliability: Choose a checker with proven reliability, as demonstrated through various testing methods and user testimonials.
- Evaluate Cost Efficiency: Balance your budget with required features, seeking tools that offer the best value for your specific requirements.
Recommended Tools and Resources
Some noteworthy AI checkers to consider include:
- ZeroGPT: Comprehensive detection with a multi-stage analysis method.
- QuillBot: Easy to use and effective for content generated by popular AI platforms.
- Grammarly: A well-established tool that merges writing assistance with AI detection features.
- GPTZero: Known for its high accuracy and user-friendliness.
Final Thoughts on AI Detection
AI detection tools are becoming indispensable as the landscape of digital content evolves. By understanding their capabilities, limitations, and the necessary steps to utilize them effectively, users can better safeguard their academic integrity and business authenticity. Whether it’s a free checker or a premium tool, ensuring that the right choices are made can help leverage the value of AI technology while upholding the principles of honest and transparent communication.