Effective Approaches for Developing an AI Strategy in Your Business
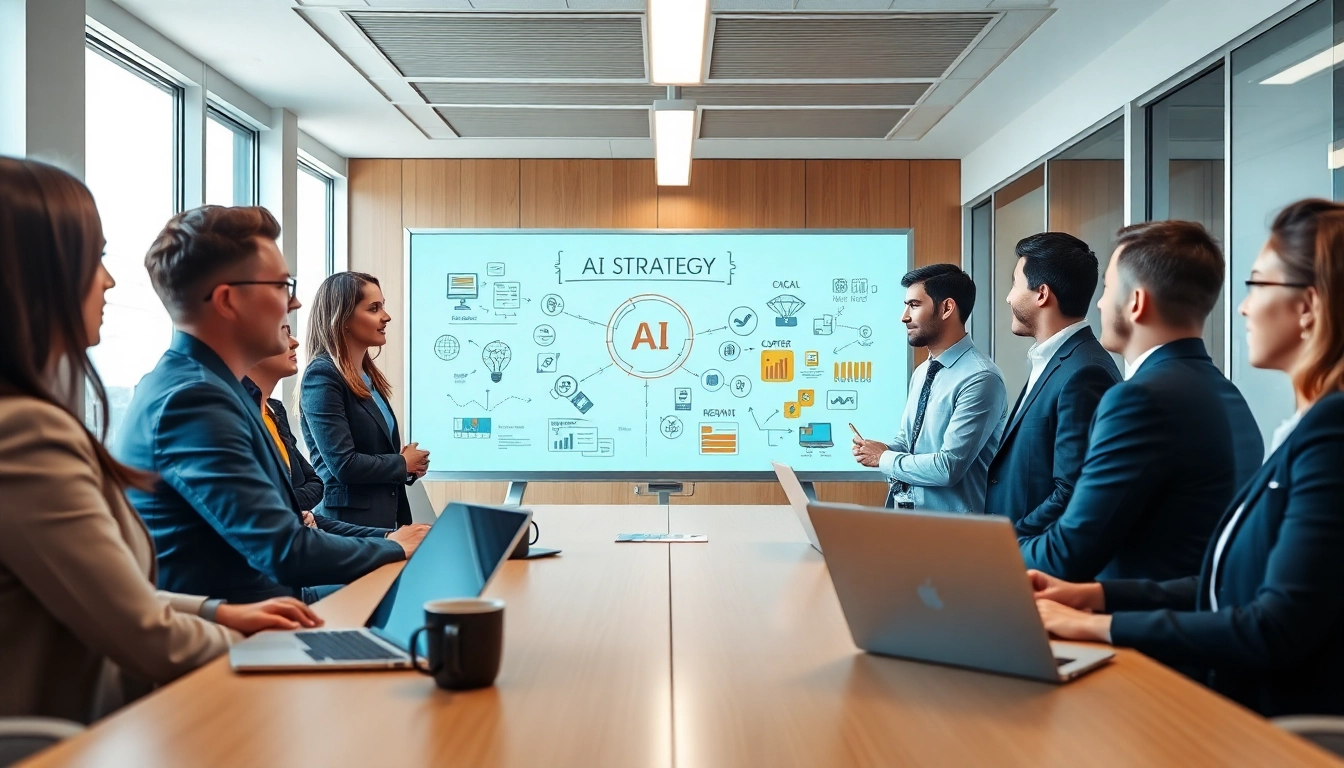
Understanding AI Strategy
Defining AI Strategy and Its Importance
In today’s rapidly evolving technological landscape, an effective ai strategy is paramount for organizations aiming to remain competitive. An AI strategy refers to the comprehensive plan that outlines how artificial intelligence can be integrated into a business’s processes to achieve specific objectives. The significance of having a robust AI strategy lies in its ability to provide clear direction, align technology initiatives with business goals and maximize the return on investment. Furthermore, organizations that adopt and adapt AI strategically can harness data-driven insights, automate processes, and enhance customer experiences, thereby catalyzing growth and innovation.
Key Components of a Successful AI Strategy
A successful AI strategy encompasses several critical components:
- Vision and Objectives: Defining clear goals for AI implementation, aligned with the overall business vision.
- Data Strategy: Implementing robust data governance frameworks to ensure quality data is available for training AI systems.
- Technology Selection: Identifying appropriate AI tools and technologies that meet the organization’s needs.
- Talent and Skills: Building a skilled workforce capable of developing, implementing, and maintaining AI initiatives.
- Ethics and Compliance: Establishing ethical guidelines and ensuring regulatory compliance pertaining to AI usage.
Integrating these components helps organizations not only implement AI technologies effectively but also leverage them for strategic decision-making and operational efficiencies.
Common Misconceptions about AI in Business
Despite the growing presence of AI in various sectors, several misconceptions surround its implementation and potential:
- AI Replaces Humans: A prevalent myth is that AI technology will entirely replace human roles. In reality, AI advancements frequently enhance human capabilities rather than replace them.
- AI is Only for Large Corporations: Many small to medium enterprises (SMEs) can also benefit from AI, adopting tailored solutions for their specific needs.
- Quick Fix: Businesses often believe that AI implementation is a quick fix for operational issues. Implementing AI requires thorough planning, testing, and continuous evaluation.
Addressing these misconceptions is key to fostering an environment conducive to innovation and effectively integrating AI strategies into business processes.
Steps to Develop Your AI Strategy
Assessing Business Objectives and Needs
The foundation of a successful AI strategy starts with a comprehensive assessment of business objectives and needs. Organizations should ask critical questions such as:
- What are our primary business challenges that AI could help solve?
- How can AI align with our long-term business goals?
- What specific processes could benefit from automation or enhancement through AI?
This assessment phase often involves collaboration among various stakeholders, ensuring that all perspectives are considered in the strategic plan.
Identifying AI Tools and Technologies
Once business needs are identified, the next step is to explore AI tools and technologies suitable for addressing those needs. Businesses should consider various factors: the size of the organization, the existing technological infrastructure, and budget constraints. Popular AI technologies that organizations might explore include:
- Machine Learning: Techniques that allow systems to learn from data and improve over time without being explicitly programmed.
- Natural Language Processing (NLP): Used for enabling machines to understand and interact in human language.
- Computer Vision: Technology that allows computers to interpret and make decisions based on visual data.
- Robotic Process Automation (RPA): Automates repetitive tasks in business processes.
Choosing the right tools hinges on aligning them with business priorities and the expertise available within the organization.
Creating a Roadmap for Implementation
A well-defined roadmap lays the groundwork for successful AI strategy execution. The roadmap should outline:
- The sequencing of AI initiatives based on priority and potential impact.
- The resources required, including budget, technology, and human capital.
- The timeline for implementation and testing phases.
- The milestones to measure progress throughout the implementation.
This structured approach ensures that the implementation process is systematic and manageable, mitigating risks associated with AI deployment.
Best Practices for AI Strategy Implementation
Engaging Stakeholders Across the Organization
Effective AI strategy implementation hinges on strong stakeholder engagement across the organization. Involving diverse teams, from IT to business units, fosters a collaborative environment where insights and expertise can be shared. Key steps include:
- Establishing a cross-functional team to oversee AI initiatives.
- Communicating the objectives and benefits of AI clearly to all stakeholders.
- Providing training sessions to promote understanding and acceptance of AI technologies.
When every team member understands and supports the AI strategy, the likelihood of successful implementation increases significantly.
Measuring Success: KPIs for Your AI Strategy
To gauge the effectiveness of an AI strategy, organizations must establish clear Key Performance Indicators (KPIs). These metrics should reflect both the performance of AI algorithms and their impact on business objectives. Commonly used KPIs include:
- Accuracy: Measuring the precision of AI outputs against expected results.
- Cost Savings: Tracking reductions in operational costs due to automation and efficiency improvements.
- Customer Satisfaction: Analyzing improvements in customer feedback and engagement due to enhanced service offerings.
- Time to Market: Evaluating the speed of implementing AI-driven solutions compared to traditional processes.
Tracking these KPIs consistently provides valuable insights for refining AI strategies and understanding their business value.
Iterating Your AI Strategy Based on Feedback
One of the fundamental aspects of an effective AI strategy is its iterative nature. Feedback loops should be established to gather insights from stakeholders and end-users about the performance of AI initiatives. Actions to consider include:
- Conducting regular review meetings to assess progress and address challenges.
- Utilizing data analytics to capture user engagement with AI systems.
- Adapting AI models based on real-time usage and feedback to optimize performance.
This agile approach enables continuous improvement and ensures that the AI strategy remains relevant and aligned with evolving organizational goals.
Examples of Successful AI Strategies
Case Study: AI Strategy in Retail
Retail giants are increasingly leveraging AI to enhance customer experiences and optimize inventory management. For instance, a well-known e-commerce platform implemented machine learning algorithms to analyze customer purchase behavior and predict inventory needs. As a result:
- The company improved its inventory turnover by 30%, reducing costs associated with overstocking.
- Customer satisfaction ratings increased due to improved product availability and personalized recommendations.
This case illustrates how an effective AI strategy can lead to substantial operational efficiencies and enhanced consumer engagement.
Case Study: AI Strategy in Healthcare
In healthcare, AI has transformed diagnostics and patient care processes. A leading healthcare provider integrated AI-driven diagnostics into its workflow. By employing natural language processing, they enhanced the accuracy of patient records analysis, leading to quicker diagnoses. This resulted in:
- An increase in diagnostic accuracy rates by 40%.
- A reduction in patient wait times by 20%, significantly improving patient experience.
This example underscores the potential of AI in advancing healthcare outcomes through precise, data-driven methodologies.
Lessons Learned from Leading Firms’ AI Strategies
Emulating best practices from successful companies can provide valuable lessons for organizations looking to implement AI strategies:
- Start Small: Leading firms often begin with pilot projects to gauge effectiveness before scaling.
- Foster a Culture of Innovation: Organizations that encourage experimentation and learning typically succeed in AI adoption.
- Focus on Data Quality: The quality of data directly affects AI accuracy, making data governance a priority.
By learning from these experiences, organizations can avoid common pitfalls and enhance their AI strategy development process.
Future Trends in AI Strategy
The Role of AI in Business Evolution
The landscape of business is constantly evolving, with AI positioned as a key driver of change. Future trends indicate that AI will play an even more significant role in:
- Automating complex decision-making processes, allowing human resources to focus on strategic planning.
- Enabling hyper-personalization in customer interactions, enhancing customer loyalty.
- Streamlining supply chain functionalities through predictive analytics and real-time data processing.
Organizations must remain agile to adapt to these evolving trends to harness AI’s full potential for business growth.
Emerging Technologies Impacting AI Strategy
As technology progresses, new innovations will shape the future of AI strategies. Emerging technologies that will likely impact AI strategy include:
- Quantum Computing: Capable of processing large datasets at unprecedented speeds, quantum computing could revolutionize AI capabilities.
- Edge Computing: Reducing latency by processing data closer to the source allows for real-time analytics and faster decision-making.
- Blockchain: Facilitating secure transactions and data sharing enhances trust and accountability in AI applications.
Understanding and integrating these technologies will be crucial for organizations striving to advance their AI initiatives.
Preparing for Future Challenges in AI Adoption
As organizations increasingly adopt AI technologies, it is essential to be proactive about potential challenges. Common concerns include:
- Data Privacy: Stricter regulations around data usage necessitate robust compliance strategies.
- Ethical Dilemmas: Addressing bias in AI systems is vital to ensure fairness and equity in AI applications.
- Skills Gap: As technology evolves, organizations must continuously invest in upskilling their workforce to maintain a competitive edge.
By anticipating these challenges, organizations can build resilience within their AI strategy, ensuring sustainable and ethical AI adoption.
Leave a Reply