Understanding Agentic AI: The Future of Autonomous Decision-Making
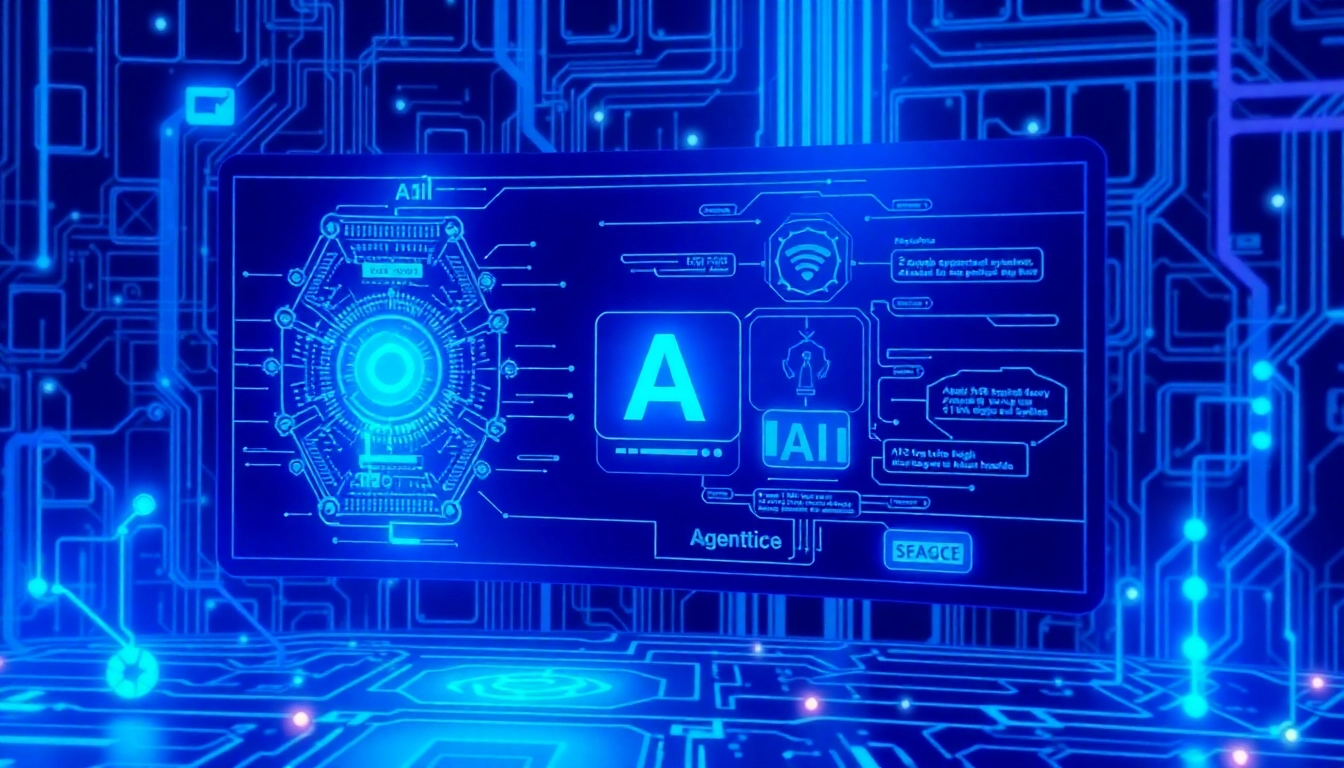
What is Agentic AI?
Agentic AI represents a transformative shift in the landscape of artificial intelligence, characterized by its capacity to operate autonomously, make decisions, and achieve complex goals without continuous human oversight. This technology employs advanced reasoning and iterative planning techniques to tackle multifaceted problems in dynamic environments. Unlike traditional AI, which often relies heavily on human input, Agentic AI systems can evaluate situations, learn from feedback, and adapt their approaches, making them highly effective across various applications.
Definition and Key Features
Agentic AI can be defined as a category of artificial intelligence systems that are designed for autonomy and decision-making. Its key features include:
- Autonomy: The ability to perform tasks and make decisions independently without human intervention.
- Adaptability: The capacity to modify behavior based on changes in the environment or task requirements.
- Learning: Utilizing machine learning algorithms to improve performance over time by recognizing patterns and outcomes.
- Multitasking: The capability to handle multiple objectives or tasks simultaneously through concurrent processing.
Comparison with Other AI Types
To appreciate the uniqueness of agentic AI, it’s essential to compare it with other forms of AI, particularly generative AI. Generative AI focuses on content creation, such as text or images, based on user prompts. It relies on human input to establish context and objectives. In contrast, agentic AI is action-oriented, emphasizing the execution of tasks and achieving predefined goals through autonomous decision-making. This distinction makes agentic AI suitable for applications that require proactive rather than reactive responses.
Moreover, while traditional AI often serves as a tool for human operators, agentic AI is akin to a co-worker or assistant capable of taking the initiative. This autonomy enables organizations to leverage AI to enhance efficiency and innovation.
Real-World Applications
Agentic AI is making substantial inroads across various industries, demonstrating its versatility and effectiveness. Some prominent real-world applications include:
- Finance: Automated trading systems that analyze market trends and make investment decisions based on real-time data.
- Healthcare: AI systems that assist in diagnosing diseases, recommending treatments, and even managing patient care autonomously.
- Transportation: Self-driving vehicles utilize agentic AI to navigate, make real-time decisions, and adjust to road conditions without human input.
- Manufacturing: Smart factories equipped with agentic AI can optimize production processes, manage inventory levels, and minimize downtime through predictive maintenance.
- Customer Service: Virtual agents equipped with agentic AI capabilities can handle inquiries, resolve issues, and escalate problems efficiently without human oversight.
How Agentic AI Works
The functionality of agentic AI stems from the integration of sophisticated technologies and methodologies that enable its autonomous operation. Understanding these processes is crucial for organizations looking to leverage this technology.
Core Technologies and Algorithms
At its core, agentic AI employs a combination of advanced algorithms, including:
- Machine Learning: Agents learn from data and past experiences, enhancing their effectiveness through iterative training. Machine learning models can be supervised, unsupervised, or reinforcement learning based on the context of application.
- Deep Learning: A subset of machine learning that uses neural networks with multiple layers to process complex data inputs, enabling agentic AI to execute sophisticated tasks like image and speech recognition.
- Natural Language Processing (NLP): Ensures that AI can understand and generate human language, facilitating seamless interaction between AI and users.
- Predictive Analytics: Leverages historical data to forecast future outcomes, which is critical for decision-making processes in dynamic environments.
Learning from Interaction
One of the hallmark features of agentic AI is its ability to learn from interactions. Each engagement provides valuable data that the AI can analyze to refine its decision-making processes. This principle operates in a feedback loop where the AI receives input, executes tasks, and subsequently evaluates the outcomes to enhance future performance. Applications in customer service are prime examples, as chatbots learn from user interactions to improve response accuracy and customer satisfaction.
Adapting to Dynamic Environments
Agentic AI’s adaptability is vital for its success in ever-changing environments. Utilizing real-time data, these systems can adjust their strategies according to new information or shifts in context. For instance, in autonomous vehicles, agentic AI continuously analyzes surrounding conditions, modifying driving patterns based on varying traffic situations, weather conditions, and pedestrian behaviors.
Benefits of Implementing Agentic AI
The integration of agentic AI into business operations can yield numerous advantages, enhancing both efficiency and productivity.
Efficiency and Cost Reduction
By automating routine tasks and processes traditionally reliant on human labor, agentic AI can significantly reduce operational costs. This efficiency not only minimizes the need for large workforces but also optimizes resource allocation, as tasks are completed more quickly and accurately. This capability is especially critical in industries like manufacturing, where precision and speed can translate to substantial financial savings.
Improved Decision-Making
Agentic AI enhances decision-making by providing data-driven insights and recommendations, removing the element of human bias. These systems can process vast amounts of information far quicker than humans, uncovering trends and patterns that may otherwise go unnoticed. For instance, in finance, AI-driven analysis can lead to better risk management strategies and investment opportunities.
Enhanced User Experience
The deployment of agentic AI often results in more personalized and responsive user experiences. In customer service applications, for instance, AI can tailor interactions based on previous customer behavior, leading to faster resolutions and greater customer satisfaction. By understanding user preferences and needs, agentic AI systems can create a more engaging and effective interaction model.
Challenges in Developing Agentic AI
While the potential for agentic AI is immense, various challenges must be addressed to fully harness its capabilities.
Ethical Considerations
As agentic AI systems become increasingly autonomous, ethical questions arise regarding accountability, decision-making, and the potential for bias in AI algorithms. Developers and organizations must prioritize ethical AI design, ensuring that systems operate fairly and transparently, and take corrective measures for any unintended biases that may emerge.
Data Privacy Issues
Agentic AI systems thrive on data, raising significant privacy concerns. The collection, storage, and usage of personal data must comply with stringent regulations like GDPR and CCPA. Organizations must implement robust data protection strategies to safeguard user information and maintain trust.
Technical Limitations
Developing effective agentic AI systems can be complicated due to technological constraints. Challenges such as algorithm bias, data quality issues, and system interoperability must be navigated to ensure successful deployment. Continuous updates and enhancements to underlying technologies are essential for overcoming these limitations and driving advancement.
The Future of Agentic AI
Looking ahead, the evolution of agentic AI poses exciting opportunities alongside inevitable challenges. Awareness of emerging trends and innovations will be critical for stakeholders across industries.
Emerging Trends and Innovations
The landscape of agentic AI is continually evolving. Current trends include:
- Increased Integration with IoT: As the Internet of Things (IoT) expands, agentic AI will find greater applications in managing connected devices and systems.
- Collaboration with humans: Future iterations of agentic AI will likely emphasize collaboration and synergy between humans and AI, maximizing the strengths of both entities.
- Enhanced Natural Language Processing: As NLP technology improves, user interactions will become increasingly seamless and intuitive, enhancing overall user engagement.
Industry Predictions
Experts predict that agentic AI will significantly influence sectors like healthcare, finance, logistics, and customer service within the next decade. As AI systems become more sophisticated, they’re expected to play a pivotal role in decision-making processes, driving innovation, and improving efficiency across these sectors. Investments in AI technologies are anticipated to accelerate as organizations recognize their substantial return on investment.
Integration into Everyday Life
The integration of agentic AI into everyday life will likely unfold gradually. From personal assistants in smart homes to AI-driven health monitoring systems, the penetration of agentic AI into personal and professional realms will reshape how individuals interact with technology. Awareness and education on the benefits and implications of agentic AI will be essential as society grapples with this paradigm shift.
Leave a Reply